Data Lakehouse
Architecture
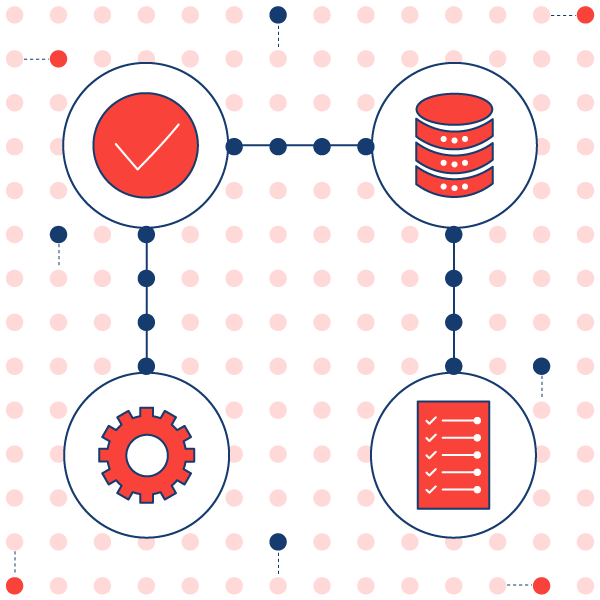
Unlock Your Data’s Potential with Lakehouse Architecture
Optimize Enterprise Data with Advanced
Lakehouse Architecture
Unified Data Architecture Framework
Centralize diverse data formats onto a single platform, streamlining overall data architecture.
Cost-Efficient Storage and Analysis
Efficiently store vast datasets and harness robust processing capabilities for comprehensive data analytics.
Robust Data Governance
Merge warehouse governance standards with lake scalability to ensure data quality, security, and regulatory compliance.
Instant Analytics for Swift Decision-Making
Enable instant analytics to facilitate swift, data-driven decisions in dynamic industries.
Scalablility and Flexibility
Dynamically adjust storage and computational resources to meet evolving data requirements.
Advanced Data Science and Machine Learning
Data lakehouses provide an efficient platform for complex data science and machine learning operations.
Collaboration Across Departments
Data lakehouses promote teamwork between IT, analytics, and business units by offering a unified data source.
Cost Reduction
Automate data processes to reduce operational expenses and eliminate redundant data systems, resulting in cost savings.
Data Lakehouse Architecture: Unifying the
Data Lake and Data Warehouse
Our expertise extends to various lake house technologies, including Amazon Redshift, Databricks, Azure Synapse Analytics, Google BigQuery, Snowflake, and MySQL HeatWave. We are committed to embracing emerging technology.
Data lakehouse architecture integrates the benefits of both data lake and data warehouse paradigms:
Data Lake
Data Lake offers flexible storage for structured, semi-structured, and unstructured data in its original format, facilitating extensive data storage.
Data Warehouse
Data Warehouse optimizes querying and analysis, with a focus on data-driven decision-making.
Key Components of a Robust Lakehouse
Architecture
Storage Infrastructure
A data lakehouse provides scalable and cost-effective data lake storage solutions.
Data Management Layer
Organize and manage data through metadata and schema management.
ACID Transactions
Ensure data integrity for concurrent operations.
Processing Framework
Manage both batch and real-time data processing, including ETL and data engine functionalities.
Governance and Security Measures
Ensure regulatory compliance, conduct data auditing, and implement robust security measures.
Query Optimization Layer
Enable SQL queries and enhance analytics through optimization techniques.
Integration Tools and APIs
Establish connections with external systems and furnish APIs for seamless development.
Advanced Analytics and Machine Learning (ML) Support
Enable sophisticated machine learning and data science operations.
Innovate with Data Lakehouse Architectures
Implementing a Data Lakehouse
Architecture: Key Stages
Planning and Strategy
- Requirement Analysis: Understand data requirements and intended use cases.
- Architecture Design: Select the appropriate technology stack and lakehouse architecture.
Infrastructure Setup
- Storage Configuration: Establish data lake storage (ex. AWS S3, Azure Blob Storage).
- Compute Resources: Provision the necessary computational resources for data processing.
Data Ingestion and Integration
- Data Sources Identification: Identify various data sources.
- Data Ingestion Pipeline Development: Build both batch and real-time data ingestion pipelines.
Data Processing and Transformation
- ETL/ELT Process Implementation: Implement data cleansing and transformation operations.
- Data Cataloging: Categorize metadata for effective data management.
Data Governance and Security
- Access Control: Configure role-based access control mechanisms.
- Compliance and Data Privacy: Ensure compliance with data privacy regulations.
Analytical and Query Tools Setup
- SQL Query Engine Configuration: Enable SQL query capabilities.
- Data Science and Machine Learning Tools: Integrate analytics and ML platforms.
User Interface and Reporting
- Dashboards and Visualization Tools: Configure tools for data visualization and reporting.
Monitoring and Maintenance
- Performance Monitoring: Implement performance monitoring tools.
- Data Quality Checks: Regularly verify and maintain data quality standards.
Success Story
40% Lower TCO with a Modern Data Analytics Platform in Azure Cloud
Our client, one of the largest and most influential chambers of commerce in North America, needed a modern analytics platform to centralize data and enable the generation of dashboard reports. Working with Adastra, the client moved their data from Excel spreadsheets to Microsoft Azure.
The new cloud analytics platform supports high volume of data ingestion while optimizing the cost and processing of information. In the new data warehouse, data is staged in an enterprise data warehouse and is extracted from the data lake 20x faster. Key benefits include:
40%
recued total cost of ownership (TCO)
3x
faster solution development
100%
clean data
Success Story
10x Increase in Productivity with an AWS Data Lake Implementation
Skylight Health Group, a healthcare services and technology company operating in the United States, needed to address challenges in consolidating their accounting data and achieve unified reporting. Adastra built a data management solution within the AWS Cloud environment that consisted of a data lake and a data warehouse.
Following the partnership with Adastra, Skylight Health Group received the following benefits:
10x
more productive analytics team
Zero
manual effort needed to produce unified and consolidated reports
Zero
infrastructure maintenance needed
Success Story
15x Faster Reporting with an AWS Data Lake Implementation
A Single
source of truth for all data
15x
faster reporting
7x
faster data processing